Beyond code: Addressing AI bias with inclusive governance
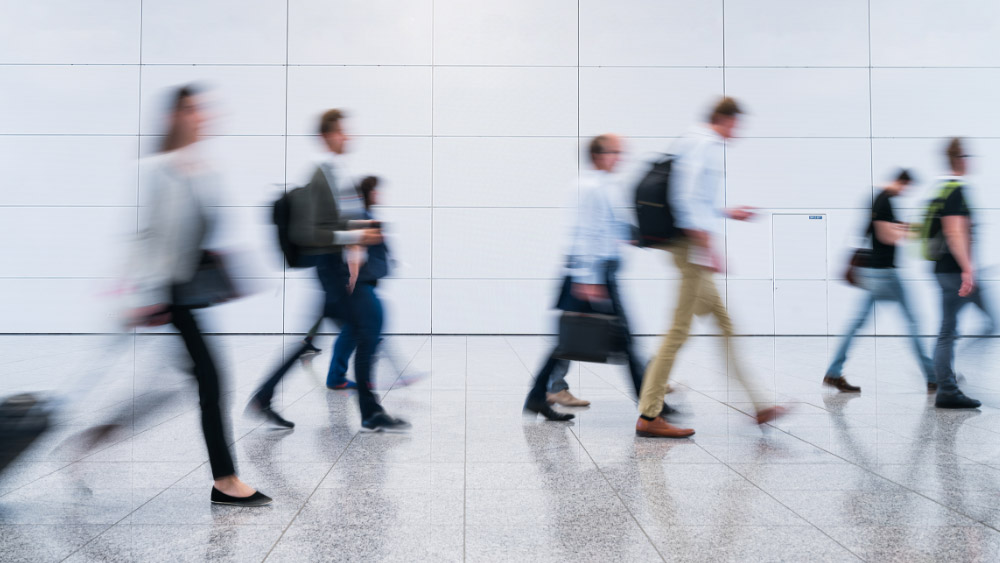
As artificial intelligence becomes increasingly embedded in our everyday lives, the conversation around AI bias and its implications has never been more urgent. Businesses are racing to leverage AI for data-driven decision-making, yet many overlook a critical issue: the very algorithms designed to enhance efficiency can perpetuate existing societal biases. Recent high-profile examples of AI bias and hallucinations, as well as reports on the lack of diversity in the tech space, have highlighted the potential pitfalls, raising alarms about the need for proper governance to protect the integrity of these systems. This article explores the complexities of AI bias, examining its roots, the consequences for businesses and society, and the essential role of diversity and governance in fostering fair and accountable AI solutions.
What is AI bias?
By now, many of us have heard of the concept of AI bias and hallucinations referred to numerous times. Normally when we talk about AI bias, we are talking about the manifestation of biased or prejudiced results in an AI algorithm due to flawed assumptions placed as part of a machine learning process. Often, the original training data is skewed by human bias, absorbed from the biases of society reflected in the data. So, algorithms can reveal and reinforce existing biases, or even create new biases where the user places trust in the distorted datasets.
In turn, this allows the AI to create ‘hallucinations’ – essentially an invention of false or contradictory sources, contexts or events presented convincingly as facts. It goes without saying that such hallucinations could have a huge impact on business decisions, as well as reputational repercussions if certain groups in society are prejudiced, or if a business ends up relying on completely fake data. Many are aware of a US case last year where a New York lawyer faced disciplinary action after referencing cases in a court hearing that did not exist. The lawyer had relied on ChatGPT to assist with legal drafting, which had resulted in hallucinations in the resulting submissions. The system produced examples of previous cases that seemed to support that lawyer’s position and arguments, all of which were fake. Other’s might recall that a high profile AI designed to assist with scientific research that suffered from so many hallucinations that the AI was shut down after 3 days. This AI was meant to summarise scientific articles and resources, but instead received backlash for the hallucinations it produced in the form of wiki articles about the history of bears in space as easily as ones about the speed of light. Most dangerously, where some hallucinations were easy to spot, many were subtly wrong in ways that would be difficult to easily identify.
And according to our latest Tech Index Report, 7 in 10 businesses are looking at development driven by AI over the next 5 years. So, what do we need to think about when thinking about AI bias?
Sources of AI bias
Many conversations in the last year around AI bias have focussed on the input or training data used to develop or teach the AI system. Advice tends to be to “understand the problem” that AI is seeking to solve, then think about buying or developing a secure AI model for that problem and being selective about training datasets, trying to make sure that such data is as diverse as possible.
And data is often a primary problem when it comes to AI bias. It may be that the data reflects pre-existing biases of society, or the data set itself was not statistically valid – either being the wrong sample, not a large enough dataset, or even that certain data is excluded.
One area where this has been particularly evident is AI developed and used as part of recruitment processes. A large online retail platform received scrutiny for this, as the AI had been training on CVs from the late-90s / early 00s, where most employees in the industry during this period were male. The AI downgraded CVs submitted by women and was ruled to be discriminatory in nature as a result. Journalists researching AI bias have reported such retrograde examples as text-to-image generation systems that overwhelmingly represent lawyers as white males, with women in a legal office setting represented as secretaries. Others cite examples of LLM predictive word generation producing results like ‘father is to doctors as mother is to… [nurse]’. Currently, AI systems haven’t fully combatted this issue, but some systems deploy embedded prompt engineering to always include a final assumption that [Job role] can be from any background, gender, race etc.
And so many of the discussions we have seen have come to the same conclusion: the AI and the human must work together. The human becomes the back stop – it is for the developer to identify hallucinations or AI bias impacting the algorithm. And with many of these AI models, the small print reads ‘AI system can make mistakes, check important info’ – so the risk is placed on the user to check the sources depicted by the AI.
Does this solve the problem in practice? We know that ‘to err is human’, and we are each impacted by unconscious bias and prejudices. While businesses are becoming more attuned to understanding that they need to look at the data sets that are feeding the AI, AI bias isn’t solely about the data.
AI developers may unintentionally inject their own unconscious biases into algorithms during design and training, and because of such biases may even fail to spot these in the outcomes of the AI model. Developers write the algorithms, choose the data used by algorithms and decide how to apply the results of the algorithms. Without diverse teams and rigorous testing, it can be too easy for people to let subtle, unconscious biases enter, which AI then automates and perpetuates. That’s why it’s so critical for the data scientists and business leads who develop and instruct AI models to test their programmes to identify problems and potential bias.
If we just look at gender, the World Economic Forum states that it will take another 132 years to achieve gender equality on a global scale. It’s 2024 report shows a circa 30% representation rate for women, and that’s across science, technology, engineering and mathematics. According to 2019 estimates from UNESCO, only 12 percent of AI researchers are women, and they “represent only six percent of software developers and are 13 times less likely to file an ICT (information, communication, and technology) patent than men.” And from an M&A investment perspective, a report by the Alan Turing Institute showed female-founded AI startups win just 2% of funding deals in the UK over the last decade, with secured funding averaging GBP1.3m a deal compared to GBP8.6m raised by all-male founder teams. If we look beyond AI used in recruitment processes and think about how it might be used to monitor productivity or performance (e.g. number of keystrokes per minute), how is this managed for employees with disabilities or a requirement for reasonable adjustments that may not feed directly into the pre-determined data set? If this is the reality of the challenge when it comes to the lack of diversity in tech resourcing, we have to ask ourselves how this manifest in the very technologies that are built. In conclusion, addressing AI bias and hallucinations requires a multifaceted approach that prioritises diversity and robust governance. As AI continues to permeate various sectors, it is crucial for businesses to recognise that the development and deployment of these technologies are not just technical challenges but social responsibilities. A diverse team brings varied perspectives that can mitigate unconscious biases, leading to more equitable AI systems.
Implementing a comprehensive governance framework, grounded in the five pillars of risk assessment – employment, technology, data protection, stakeholder engagement, and litigation – enables organisations to systematically identify and address potential biases throughout the AI lifecycle. By fostering inclusive environments and prioritising ethical AI practices, businesses can ensure that their technologies not only drive innovation but also reflect the diverse society they serve. As we move forward, it’s imperative for leaders in technology and business to collaborate in creating AI systems that uphold fairness and transparency. The future of AI should not just be about efficiency and profit but about empowering all individuals and communities. By embracing diversity and committing to rigorous governance, we can harness the transformative power of AI to create a more equitable and just world.